Introduction: Charting the Future of AI in Technical Content
In the rapidly evolving landscape of AI-driven technical content, the integration of advanced technologies like Retrieval-Augmented Generation (RAG) and semantic search has already started reshaping how organizations manage their documentation. In our first two blog posts, we explored how RAG enhances the accuracy and relevance of AI responses and how semantic search elevates content retrieval by understanding user intent more effectively.
However, the journey doesn't stop here. As AI continues to advance, so do the methodologies we employ to optimize content delivery. In this final post of our series, we delve into the future of AI in technical documentation by introducing GraphRAG – a cutting-edge approach that combines knowledge graphs with RAG, promising even greater accuracy, relevance, and flexibility in AI-driven applications.
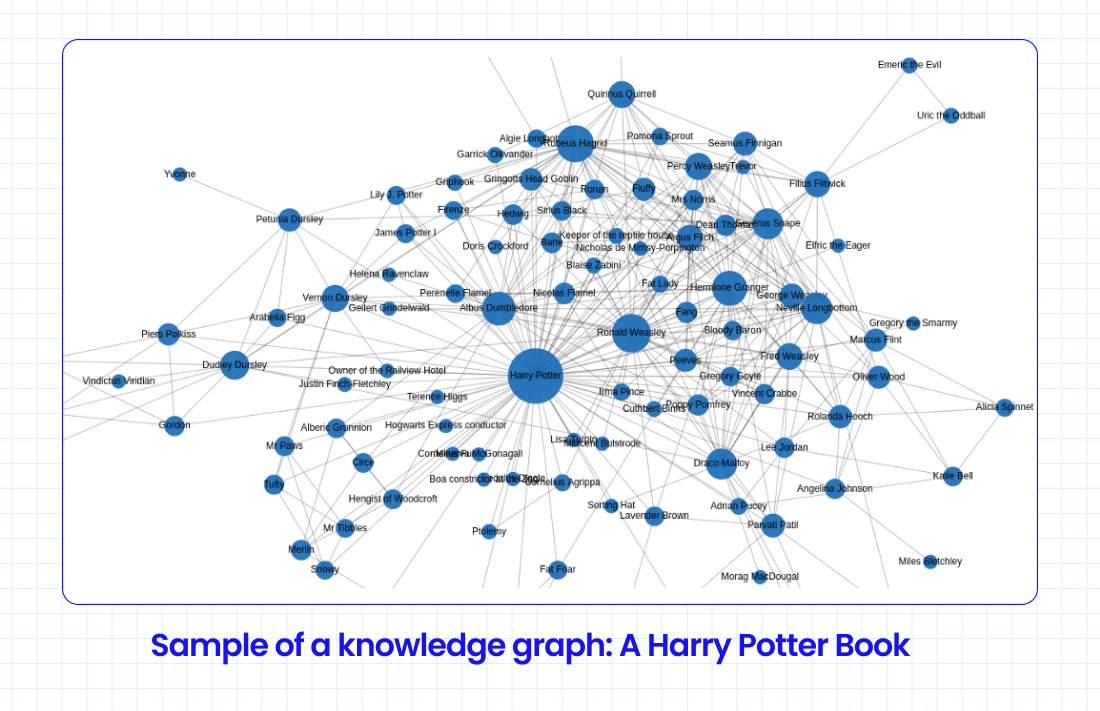
Understanding Knowledge Graphs: Building Connections Beyond Text
What Is a Knowledge Graph?
To appreciate the potential of GraphRAG, it's essential first to understand what a knowledge graph is. At its core, a graph is a collection of nodes (representing entities) connected by edges (representing relationships). This structure can represent a wide array of data, from social networks to molecular structures.
A knowledge graph, however, takes this concept further by structuring data in a way that reflects real-world relationships and hierarchies. For example, in film, a knowledge graph can represent the relationships between different movies, genres, actors, directors, and even the themes they explore. This allows for a richer understanding of how various elements of movies are interconnected.
How Knowledge Graphs Differ from Traditional Indexing and Vector Databases
Traditional indexing systems focus on finding documents based on keyword matches. This approach works well for basic searches but may miss the deeper connections between different pieces of content.
A vector database, on the other hand, stores information as vectors – mathematical representations of data that capture the meaning and context of content. When a user performs a search, the system compares these vectors to find content that is similar in meaning, even if the exact words don't match. This is more advanced than keyword searches but still has limitations, especially with complex or interconnected information.
Knowledge graphs offer a more powerful approach by representing data as a network of interconnected nodes (pieces of content) and edges (relationships between them). This structure allows the AI to understand and navigate the rich connections between different topics, leading to more accurate and context-aware results.
Advantages of Knowledge Graphs in Technical Documentation
In the technical content domain, knowledge graphs excel by creating meaningful connections between different pieces of content. They allow AI applications to navigate through these connections, leading to more accurate and contextually relevant responses. This capability is particularly valuable when dealing with large volumes of complex, interrelated documentation where simple keyword or vector searches might miss the nuances of user intent.
Introducing GraphRAG: The Next Evolution of AI-Driven Content
What Is GraphRAG?
GraphRAG is the next evolution of Retrieval-Augmented Generation, where knowledge graphs are integrated into the RAG framework to enhance the retrieval and generation process. In traditional RAG, the retrieval phase involves searching through documents or embeddings to find the most relevant information, which is then used to generate a response. GraphRAG builds on this by using a knowledge graph to guide the retrieval process more intelligently, leveraging the relationships between content pieces to find the most relevant and context-aware information.
The Basic Flow of GraphRAG in AI Applications
Let’s break down how GraphRAG operates:
- User Query: The process begins when a user enters a search query or sends a message in a search application.
- Graph Search: Instead of a simple keyword or vector search, the application runs a search on the knowledge graph to identify the initial set of relevant nodes.
- Graph Traversal: The application then uses a strategy to traverse the graph from these initial nodes, retrieving relevant information from selected nodes.
- RAG Process: The retrieved information is used to generate a response, ensuring that the AI output is not only accurate but also deeply informed by the interconnected knowledge within the graph.
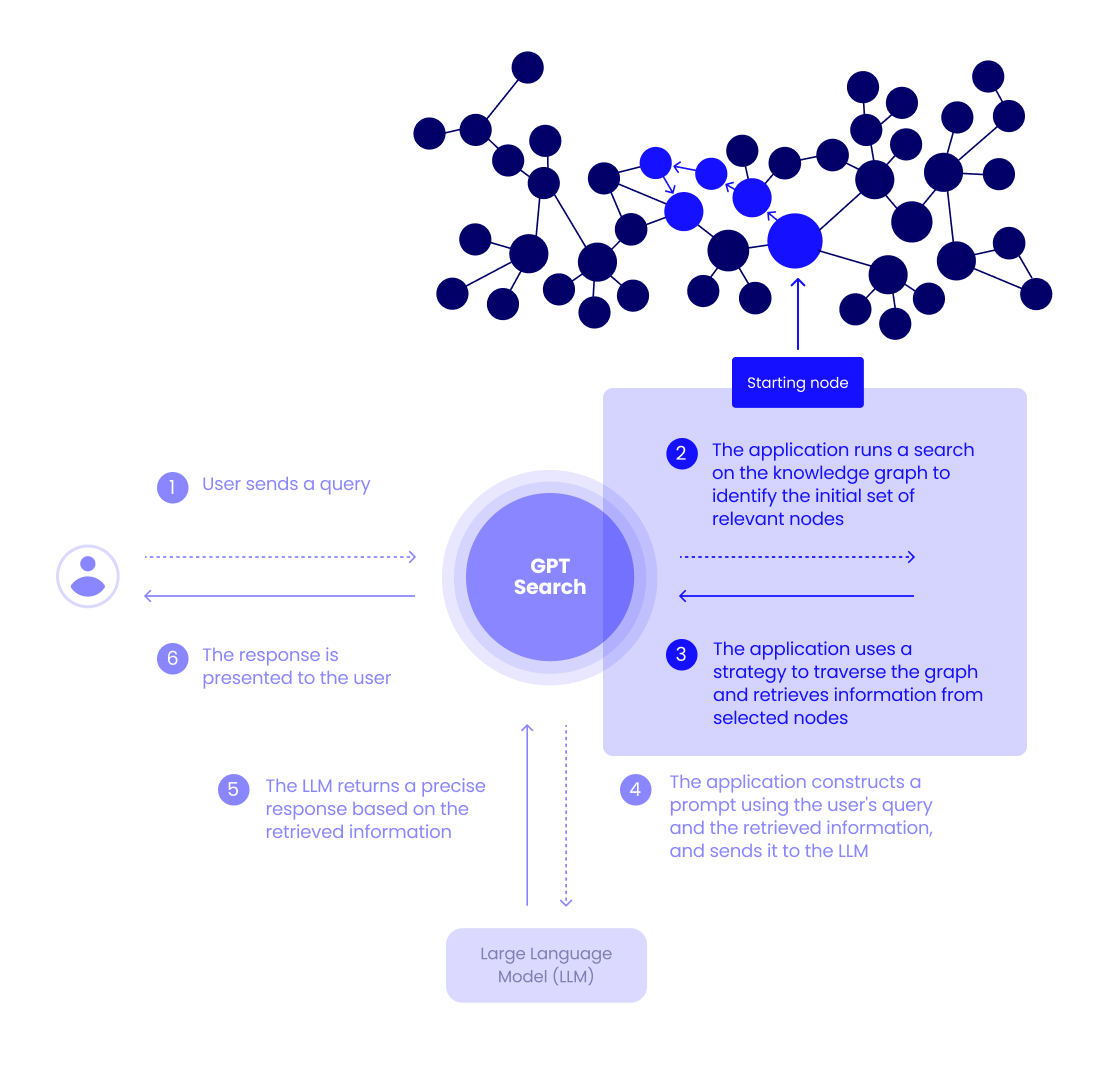
This basic flow provides an overview of how GraphRAG functions within AI applications. For a deeper technical understanding, we provide below a detailed flow, including the mechanics of GraphRAG and how it leverages knowledge graphs to enhance AI-driven content generation.
The Benefits of GraphRAG for Technical Documentation
Higher Accuracy Through Richer Connections
By integrating a knowledge graph, GraphRAG enhances accuracy while taking into account the intricate relationships between various content pieces. Unlike traditional RAG, which retrieves content based on isolated semantic similarity, GraphRAG considers the broader context, leading to more relevant results. This is especially crucial in technical documentation, where the precision of information can significantly impact the user’s ability to complete tasks or solve problems.
Creating Meaningful Connections Between Topics
One of the key advantages of GraphRAG is its ability to form meaningful connections between different topics. For example, if a technical document mentions a specific product feature in multiple contexts, a knowledge graph can link these mentions together, helping the AI application understand the broader implications and relationships between them. This additional layer of information contributes to higher relevance in the generated responses.
Flexible Retrieval Strategies for Optimal Results
GraphRAG’s use of knowledge graphs allows for more flexible strategies in retrieving content. Instead of relying solely on vector search with K-Nearest Neighbors (KNN) to find the best matches, GraphRAG can employ various strategies to pull in the most relevant content. For example, an AI application may prioritize nodes with the most direct connections to the user’s query or those that form a more comprehensive path through related documents. This flexibility ensures that the retrieval process can be tailored to deliver the best possible results in any given scenario.
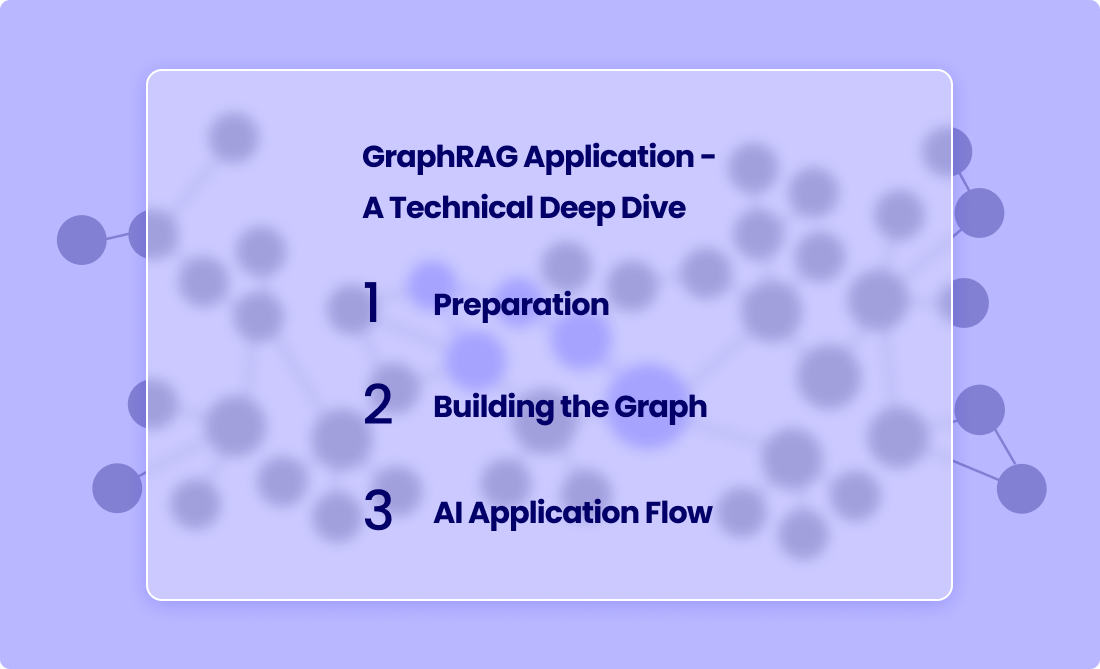
Conclusion: The Future of Technical Documentation with GraphRAG
It's becoming clear that the integration of AI in technical documentation is not just a trend but a significant leap forward in how organizations manage and deliver content. GraphRAG represents the next frontier in this evolution, offering a powerful tool to create more accurate, relevant, and context-aware AI applications that better address users' needs, allowing them to easily use technical documentation and reduce support costs.
By combining the strengths of knowledge graphs with the proven capabilities of RAG, organizations can elevate their technical content management to new heights. Whether you're enhancing existing AI-driven tools or developing new applications from the ground up, GraphRAG provides the foundation for a smarter, more connected future in documentation.
As AI continues to evolve, so will the methods we use to harness its potential. GraphRAG is just the beginning, and the possibilities for innovation in technical documentation are limitless. Stay ahead of the curve by embracing these advanced technologies and preparing your organization for the next wave of AI-driven transformation.